Collaborating IRCN principal investigators
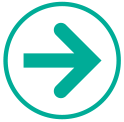
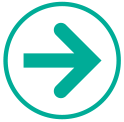
Cognitive Developmental Robotics Lab Staff
Project Professor | Yukie Nagai |
---|
Research
How do humans acquire intelligence? One approach to addressing this fundamental question is to create robots that learn and develop like humans. We design neural network models and robots inspired by the human brain and body, with the aim of understanding the principles of intelligence. A particular focus of our research is on predictive processing in the brain. The brain integrates bottom-up sensory signals with top-down predictions to minimize prediction errors through perception and action. Through the design of neural networks based on this theory, we are investigating whether the temporal continuity and individual diversity in development can be consistently explained by predictive processing. Furthermore, we apply the knowledge obtained to develop assistive technologies for individuals with developmental disorders. By collaborating with researchers who have developmental disorders, we aim to elucidate the underlying mechanisms of social difficulties.
Publication list
- Philippsen A, Tsuji S, and Nagai Y: Simulating Developmental and Individual Differences of Drawing Behavior in Children Using a Predictive Coding Model. Frontiers in Neurorobotics, 16:856184, 2022.
- Seker M Y, Ahmetoglu A, Nagai Y, Asada M, Oztop E, and Ugur E: Imitation and mirror systems in robots through Deep Modality Blending Networks. Neural Networks, 146:22-35, 2022.
- Nagai Y: Predictive learning: its key role in early cognitive development. Philosophical Transactions of the Royal Society B: Biological Sciences, 374(1771):20180030, 2019.
- Horii T, Nagai Y, and Asada M: Modeling Development of Multimodal Emotion Perception Guided by Tactile Dominance and Perceptual Improvement. IEEE Transactions on Cognitive and Developmental Systems, 10(3):762-775, 2018.
- Baraglia J, Nagai Y, and Asada M: Emergence of Altruistic Behavior Through the Minimization of Prediction Error. IEEE Transactions on Cognitive and Developmental Systems, 8(3):141-151, 2016.
Contact
office@developmental-robotics.jp
Predictive and Creative Brain Lab Staff
Associate Prof. | Zenas C. Chao |
---|
Research
Large-Scale Neuronal Networks in the Predictive and Creative Brain
Creative Brain Predictive coding theory proposes that the brain actively generates predictions about incoming sensory information to create an understanding of the external world. This is achieved by a hierarchical and bidirectional cascade of large-scale cortical signaling in order to minimize overall prediction errors. This theoretical framework provides a unified model for both perception and action and holds promise for shedding light on psychiatric disorders characterized by disturbances in prediction or error signaling, such as autism, schizophrenia, and ADHD. My current research interests are twofold: (1) validating the theory by pinpointing brain signals that underlie predictions and prediction errors at different hierarchical levels, and (2) extending the theory's applicability beyond perception and action, encompassing domains such as internal thought processes and creativity. Enhancing our understanding of how predictive coding operates across various hierarchies and sensory modalities, as well as how information from diverse spatial and temporal scales merges into a comprehensive thought, holds the potential to catalyze progress in neuromorphic engineering and propel the quest for neural markers crucial in forecasting and diagnosing brain disorders.
Projects
- Investigation of Predictive Coding Circuits: Our objective is to elucidate the intricate networks of microcircuits and macrocircuits integral to predictive coding across various hierarchies and sensory domains. This work extends across species — from rodents and marmosets to humans — and integrates experimental findings from these diverse subjects with theoretical modeling to forge comprehensive insights.
- Neural Markers for Prediction-Related Psychiatric Disorders: A pivotal aspect of our work involves pinpointing specific neural markers that correlate with psychiatric disorders linked to prediction anomalies, such as autism spectrum disorder and schizophrenia. Identifying these markers is crucial for understanding the neural foundations of these conditions and could lead to more targeted interventions.
- Understanding Predictive Coding in Creativity: Our research also explores the role of predictive coding in the realm of creative potential, specifically its capacity to foster the generation of innovative ideas for future tasks. Through this exploration, we aim to illuminate the cognitive mechanisms that underpin creativity, offering new perspectives on this complex process.
- Development of a Creativity Augmentation System for Real-World Applications: One ambition of our work is to create a closed-loop system designed to augment creative potential in practical, real-world settings. Such a system promises to revolutionize fields that depend heavily on creative problem-solving, by offering new tools and methodologies to enhance innovation.
Publication list
- Kern, F. B., & Chao, Z. C. (2023). Short-term neuronal and synaptic plasticity act in synergy for deviance detection in spiking networks. PLOS Computational Biology, 19(10), e1011554.
- Chao, Z. C., Huang, Y. T., & Wu, C. T. (2022). A quantitative model reveals a frequency ordering of prediction and prediction-error signals in the human brain. Communications Biology, 5(1), 1076.
- Chao, Z. C., Dillon, D. G., Liu, Y. H., Barrick, E. M., & Wu, C. T. (2022). Altered coordination between frontal delta and parietal alpha networks underlies anhedonia and depressive rumination in major depressive disorder. Journal of Psychiatry and Neuroscience, 47(6), E367-E378.
- Chao, Z. C., Takaura, K., Wang, L., Fujii, N., & Dehaene, S. (2018). Large-scale cortical networks for hierarchical prediction and prediction error in the primate brain. Neuron, 100(5), 1252-1266.
- Chao, Z. C., Nagasaka, Y., & Fujii, N. (2015). Cortical network architecture for context processing in primate brain. Elife, 4, e06121.
Contact
zenas.c.chao@ircn.jp